Recombination Operators
Recombination (crossover) is a fundamental genetic algorithm operator that combines the genetic information of two parents to generate new offspring. The choice of a recombination operator depends on the chromosome representation (binary, real-valued, or permutation-based) and the nature of the optimization problem.
Understanding Recombination
Recombination operators promote diversity and exploration in the solution space. They allow offspring to inherit traits from both parents, which can lead to better solutions over generations.
Below are some common recombination techniques used in genetic algorithms:
Binary Chromosomes: Techniques like One-Point Crossover and Uniform Crossover are well-suited for binary representations, where each gene is a bit (0 or 1).
Real-Valued Chromosomes: Methods such as Byte One Point Crossover, Arithmetic Crossover and BLX-Alpha Crossover facilitate exploration in continuous domains.
Permutation-Based Chromosomes: Operators like PMX (Partially Matched Crossover) ensure valid offspring while preserving order relationships in sequencing problems.
Recombination Examples
One-Point Crossover
One-Point Crossover is one of the simplest and most widely used techniques for binary chromosomes. A random crossover point is selected, and segments from the parents are swapped to create offspring.
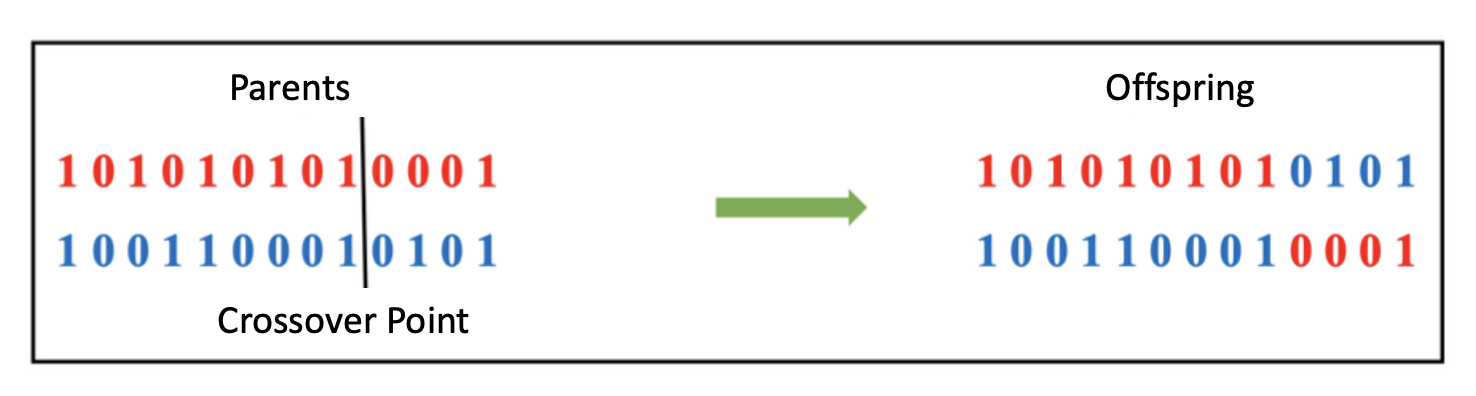
Figure 1: An example of One-Point Crossover.
Two-Point Crossover
Two-Point Crossover extends the idea of One-Point Crossover by selecting two random crossover points. The segment between the two points is swapped between the parents, producing offspring with potentially more diverse genetic combinations.
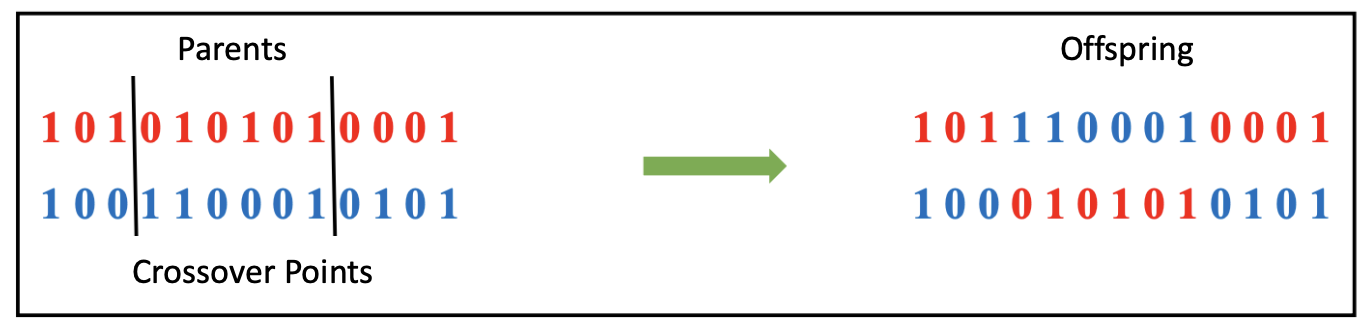
Figure 2: An example of Two-Point Crossover.
Uniform Crossover
In Uniform Crossover, a mask composed of bits is determined over the length of the chromosome. These bits, which take the value 0 or 1, specify which parent the gene for the offspring will be chosen from. Bits with the value 1 are distributed uniformly with a probability of 0.5.
For example, as illustrated below, bits in the mask with a 0 value indicate that the gene will be selected from Parent X, while bits with a 1 value indicate that the gene will be selected from Parent Y. The reverse process is applied to produce the second offspring.
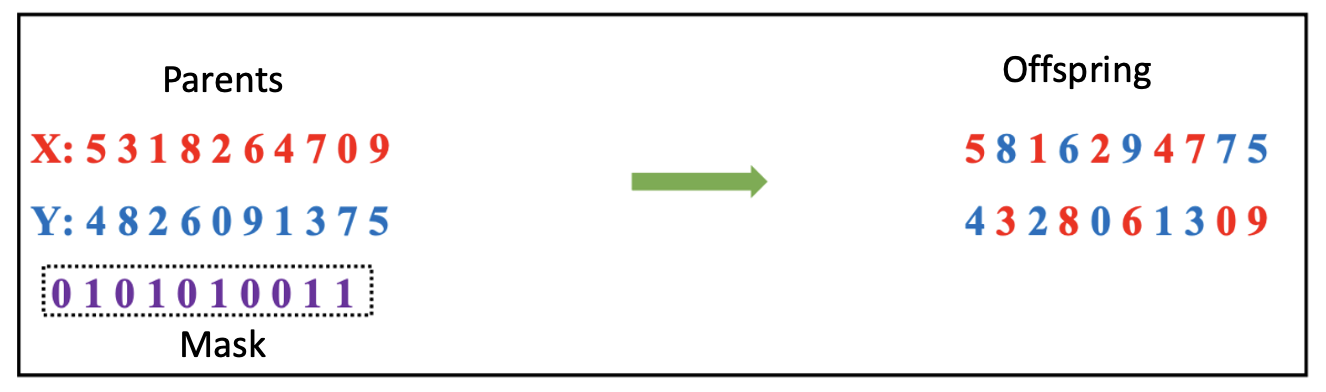
Figure 3: An example of Uniform Crossover.
API References
The following sections provide detailed documentation for the recombination operators available in the pycellga.recombination package.
Arithmetic Crossover
- class ArithmeticCrossover(parents: list, problem: AbstractProblem)[source]
Bases:
RecombinationOperator
ArithmeticCrossover performs an arithmetic crossover operation on a pair of parent individuals to produce offspring individuals.
- Parameters:
parents (list) – A list containing two parent individuals.
problem (AbstractProblem) – The problem instance that provides the fitness function.
- __init__(parents: list, problem: AbstractProblem)[source]
Initialize the ArithmeticCrossover object.
- Parameters:
parents (list) – A list containing two parent individuals.
problem (AbstractProblem) – The problem instance that provides the fitness function.
- get_recombinations() List[Individual] [source]
Perform the arithmetic crossover on the parent individuals to produce offspring.
- Returns:
A list containing the offspring individuals.
- Return type:
List[Individual]
BLX-Alpha Crossover
- class BlxalphaCrossover(parents: list, problem: AbstractProblem)[source]
Bases:
RecombinationOperator
BlxalphaCrossover performs BLX-alpha crossover on a pair of parent individuals to produce offspring individuals.
- Parameters:
parents (list) – A list containing two parent individuals.
problem (AbstractProblem) – The problem instance that provides the fitness function.
- __init__(parents: list, problem: AbstractProblem)[source]
Initialize the BlxalphaCrossover object.
- Parameters:
parents (list) – A list containing two parent individuals.
problem (AbstractProblem) – The problem instance that provides the fitness function.
- combine(p1: Individual, p2: Individual, locationsource: Individual) Individual [source]
Combine two parent individuals using BLX-alpha crossover to produce a single offspring.
- Parameters:
p1 (Individual) – The first parent individual.
p2 (Individual) – The second parent individual.
locationsource (Individual) – The individual from which to copy positional information for the offspring.
- Returns:
The resulting offspring individual.
- Return type:
- get_recombinations() List[Individual] [source]
Perform the BLX-alpha crossover on the parent individuals to produce offspring.
- Returns:
A list containing the offspring individuals.
- Return type:
List[Individual]
Byte One-Point Crossover
- class ByteOnePointCrossover(parents: list, problem: AbstractProblem)[source]
Bases:
RecombinationOperator
ByteOnePointCrossover operator defined in (Satman, 2013). ByteOnePointCrossover performs a one-point crossover at the byte level on a pair of parent individuals to produce offspring individuals.
- Parameters:
parents (list) – A list containing two parent individuals.
problem (AbstractProblem) – The problem instance that provides the fitness function.
- __init__(parents: list, problem: AbstractProblem)[source]
Initialize the ByteOnePointCrossover object.
- Parameters:
parents (list) – A list containing two parent individuals.
problem (AbstractProblem) – The problem instance that provides the fitness function.
- get_recombinations() List[Individual] [source]
Perform the one-point crossover on the parent individuals at the byte level to produce offspring.
- Returns:
A list containing the offspring individuals.
- Return type:
List[Individual]
Byte Uniform Crossover
- class ByteUniformCrossover(parents: list, problem: AbstractProblem)[source]
Bases:
RecombinationOperator
ByteUniformCrossover operator defined in (Satman, 2013). ByteUniformCrossover performs a uniform crossover at the byte level on a pair of parent individuals to produce offspring individuals.
- Parameters:
parents (list) – A list containing two parent individuals.
problem (AbstractProblem) – The problem instance that provides the fitness function.
- __init__(parents: list, problem: AbstractProblem)[source]
Initialize the ByteUniformCrossover object.
- Parameters:
parents (list) – A list containing two parent individuals.
problem (AbstractProblem) – The problem instance that provides the fitness function.
- combine(p1: Individual, p2: Individual, locationsource: Individual) Individual [source]
Combine two parent individuals using uniform crossover at the byte level to produce a single offspring.
- Parameters:
p1 (Individual) – The first parent individual.
p2 (Individual) – The second parent individual.
locationsource (Individual) – The individual from which to copy positional information for the offspring.
- Returns:
The resulting offspring individual.
- Return type:
- get_recombinations() List[Individual] [source]
Perform the uniform crossover on the parent individuals to produce offspring.
- Returns:
A list containing the offspring individuals.
- Return type:
List[Individual]
Flat Crossover
- class FlatCrossover(parents: list, problem: AbstractProblem)[source]
Bases:
RecombinationOperator
FlatCrossover performs a flat crossover on a pair of parent individuals to produce offspring individuals.
- Parameters:
parents (list) – A list containing two parent individuals.
problem (AbstractProblem) – The problem instance that provides the fitness function.
- __init__(parents: list, problem: AbstractProblem)[source]
Initialize the FlatCrossover object.
- Parameters:
parents (list) – A list containing two parent individuals.
problem (AbstractProblem) – The problem instance that provides the fitness function.
- combine(p1: Individual, p2: Individual, locationsource: Individual) Individual [source]
Combine two parent individuals using flat crossover to produce a single offspring.
- Parameters:
p1 (Individual) – The first parent individual.
p2 (Individual) – The second parent individual.
locationsource (Individual) – The individual from which to copy positional information for the offspring.
- Returns:
The resulting offspring individual.
- Return type:
- get_recombinations() List[Individual] [source]
Perform the flat crossover on the parent individuals to produce offspring.
- Returns:
A list containing the offspring individuals.
- Return type:
List[Individual]
Linear Crossover
- class LinearCrossover(parents: list, problem: AbstractProblem)[source]
Bases:
RecombinationOperator
LinearCrossover performs a linear crossover on a pair of parent individuals to produce offspring individuals.
- Parameters:
parents (list) – A list containing two parent individuals.
problem (AbstractProblem) – The problem instance that provides the fitness function.
- __init__(parents: list, problem: AbstractProblem)[source]
Initialize the LinearCrossover object.
- Parameters:
parents (list) – A list containing two parent individuals.
problem (AbstractProblem) – The problem instance that provides the fitness function.
- combine(p1: Individual, p2: Individual, locationsource: Individual) Individual [source]
Combine two parent individuals using linear crossover to produce a single offspring.
- Parameters:
p1 (Individual) – The first parent individual.
p2 (Individual) – The second parent individual.
locationsource (Individual) – The individual from which to copy positional information for the offspring.
- Returns:
The resulting offspring individual.
- Return type:
- get_recombinations() List[Individual] [source]
Perform the linear crossover on the parent individuals to produce offspring.
- Returns:
A list containing the offspring individuals.
- Return type:
List[Individual]
One-Point Crossover
- class OnePointCrossover(parents: list, problem: AbstractProblem)[source]
Bases:
RecombinationOperator
OnePointCrossover performs a one-point crossover on a pair of parent individuals to produce offspring individuals.
- Parameters:
parents (list) – A list containing two parent individuals.
problem (AbstractProblem) – The problem instance that provides the fitness function.
- __init__(parents: list, problem: AbstractProblem)[source]
Initialize the OnePointCrossover object.
- Parameters:
parents (list) – A list containing two parent individuals.
problem (AbstractProblem) – The problem instance that provides the fitness function.
- get_recombinations() List[Individual] [source]
Perform the one-point crossover on the parent individuals to produce offspring.
- Returns:
A list containing the offspring individuals.
- Return type:
List[Individual]
Partially Matched Crossover (PMX)
- class PMXCrossover(parents: list, problem: AbstractProblem)[source]
Bases:
RecombinationOperator
PMXCrossover performs Partially Mapped Crossover (PMX) on a pair of parent individuals to produce offspring individuals.
- Parameters:
parents (list) – A list containing two parent individuals.
problem (AbstractProblem) – The problem instance that provides the fitness function.
- __init__(parents: list, problem: AbstractProblem)[source]
Initialize the PMXCrossover object.
- Parameters:
parents (list) – A list containing two parent individuals.
problem (AbstractProblem) – The problem instance that provides the fitness function.
- get_recombinations() List[Individual] [source]
Perform the PMX crossover on the parent individuals to produce offspring.
- Returns:
A list containing the offspring individuals.
- Return type:
List[Individual]
Two-Point Crossover
- class TwoPointCrossover(parents: list, problem: AbstractProblem)[source]
Bases:
RecombinationOperator
TwoPointCrossover performs a two-point crossover on a pair of parent individuals to produce offspring individuals.
- Parameters:
parents (list) – A list containing two parent individuals.
problem (AbstractProblem) – The problem instance that provides the fitness function.
- __init__(parents: list, problem: AbstractProblem)[source]
Initialize the TwoPointCrossover object.
- Parameters:
parents (list) – A list containing two parent individuals.
problem (AbstractProblem) – The problem instance that provides the fitness function.
- get_recombinations() List[Individual] [source]
Perform the two-point crossover on the parent individuals to produce offspring.
- Returns:
A list containing the offspring individuals.
- Return type:
List[Individual]
Unfair Average Crossover
- class UnfairAvarageCrossover(parents: list, problem: AbstractProblem)[source]
Bases:
RecombinationOperator
UnfairAvarageCrossover performs an unfair average crossover on a pair of parent individuals to produce offspring individuals.
- Parameters:
parents (list) – A list containing two parent individuals.
problem (AbstractProblem) – The problem instance that provides the fitness function.
- __init__(parents: list, problem: AbstractProblem)[source]
Initialize the UnfairAvarageCrossover object.
- Parameters:
parents (list) – A list containing two parent individuals.
problem (AbstractProblem) – The problem instance that provides the fitness function.
- get_recombinations() List[Individual] [source]
Perform the unfair average crossover on the parent individuals to produce offspring.
- Returns:
A list containing the offspring individuals.
- Return type:
List[Individual]
Uniform Crossover
- class UniformCrossover(parents: list, problem: AbstractProblem)[source]
Bases:
RecombinationOperator
UniformCrossover performs a uniform crossover on a pair of parent individuals to produce offspring individuals.
- Parameters:
parents (list) – A list containing two parent individuals.
problem (AbstractProblem) – The problem instance that provides the fitness function.
- __init__(parents: list, problem: AbstractProblem)[source]
Initialize the UniformCrossover object.
- Parameters:
parents (list) – A list containing two parent individuals.
problem (AbstractProblem) – The problem instance that provides the fitness function.
- combine(p1: Individual, p2: Individual, locationsource: Individual) Individual [source]
Combine two parent individuals using uniform crossover to produce a single offspring.
- Parameters:
p1 (Individual) – The first parent individual.
p2 (Individual) – The second parent individual.
locationsource (Individual) – The individual from which to copy positional information for the offspring.
- Returns:
The resulting offspring individual.
- Return type:
- get_recombinations() List[Individual] [source]
Perform the uniform crossover on the parent individuals to produce offspring.
- Returns:
A list containing the offspring individuals.
- Return type:
List[Individual]